Video Clip Source: Tom Davidson, Senior Researcher at Open Philanthropy, on the Future Of Life Podcast
Video Clip Context: This clip is the best I’ve seen at breaking down the rate of evolution of the various components that go into AI’s overall rate of progress.
So, Elon Musk said this last week:
This may be Musk's boldest tweet yet, and it has received surprisingly little fanfare.
If it’s true, then we are about to see an almost unfathomable shift in human society. Therefore, we should make decisions in our lives and society very, very differently.
So what should we make of it?
Evidence that Musk is becoming more and more unhinged?
As a serious prediction by one of the world’s top AI experts?
An attempt to use his platform to raise awareness for the urgency of AI Safety?
A combo of all three?
I, for one, take his prediction very seriously. And I’m not alone. Most experts who have deeply researched AI timelines assign a surprisingly high probability that AI will progress way faster than people think.
This article will provide you with 100+ hours of research I’ve done on AI timelines so you can make up your own mind.
If you’re at all interested in AI, this timeline topic is arguably the first thing you should understand before you make a strategy for your future…
The single most important thing to understand about AI is how fast it is moving.
Dario Amodei, CEO of prominent AI company Anthropic (creator of Claude),
speaking to the Senate The Subcommittee on Privacy, Technology, and the Law
Author Note #1
This post is a continuation of a series on the predictable parts of AI’s future. The first two articles are:
Free subscribers get the whole post. Paid subscribers get six additional resources to go deeper on the topic.
Let’s jump in….
The Article
Over the last few months, I’ve spent 100+ hours refining my mental model of how the future of AI might play out. More specifically, I followed the steps below:
Identified more top experts (researchers, philosophers, entrepreneurs, prompters, engineers, journalists)
Understood the conflicts between different camps (so I could understand the pros/cons of each perspective without getting sucked into an ideology)
Consumed the top ideas (articles, surveys, studies, speeches, interviews, etc)
Explored AI using relevant mental models (exponential trends, first principles thinking, second-order effects, productivity chain, inflection points, scenario planning, probabilistic thinking, risk of ruin, hype cycles, dialectical thinking, Kubler-Ross grief cycle, systems theory, weak signals, paradigm shifts, etc)
Mapped out different scenarios of the future (including probabilities, implications, and root causes)
Extracted and combined expert ideas/predictions/models into my own larger model
Looked for counterarguments (and stress-tested my opinions)
Rethought my life in a world of advanced AI (from first principles)
Created a strategy (that takes into account many different possibilities)
Started writing posts like today’s post to clarify and test my thinking (Explanation Effect)
I’ve spent so much time on AI because it’s not only the most important invention in human history but also the fastest-growing. More so, it just passed a crucial inflection point. In roughly the past year, it suddenly became widely available, useful, and easy to use with the release of ChatGPT.
And, unless something radically unexpected happens, it will profoundly impact everyone in the coming years—likely starting this year with the release of GPT-5.
Like the month before COVID exploded in February 2020, we’re in the calm before the storm.
The Benefits Of Building Your Own Model Of AI’s Future
As I’ve spent more time creating a mental model, I notice myself shifting from overwhelm to clarity to conviction.
At first, AI news was overwhelming. I didn’t have a way to contextualize announcements of new demos and technology. I couldn’t separate hype from reality or important from non-important. It felt like I was trying to organize my clothes without having a dresser, a closet, or hangers.
But as I used mental models and then constructed my own, it felt like I suddenly had a very well-organized closet. I could contextualize news and update my model if any news contradicted or added new insights.
With this model, I started to think more strategically:
Strategy. I thought through the risks and opportunities that will arise from AI. Changed my whole business strategy (newsletter format, newsletter topics, upcoming course).
Workflow. I started to reinvent my workflow around AI. I identified the key parts of my workflow, and I’m systematically turning each part into a GPT.
Financial Investing. The stock market hasn’t priced in the potential of AGI. Therefore, I started to research what companies will most benefit from AGI.
Time Investment Into AI. As I’ve gained further conviction about AI's transformative effects, I’ve cleared more of my schedule to focus on it.
Not only that, I felt less stressed and more in control. Rather than AI news being completely shocking, disorienting, and uncertain, it has become more predictable. And I’m not alone. Research shows that proactively thinking about and preparing for future scenarios is good for your mental health. The video below from futurist and researcher Jane McGonigal explains:
Source: How To Think Like A Futurist & Be Ready For Anything | Jane McGonigal | EDU2022
Beyond these reasons, there was a deeper reason I have been so deliberate about AI…
The Deeper Reason Why I Spent 100+ Hours Researching The Model In This Article
Technology adoption is one of the fundamental underappreciated decisions we make as knowledge workers:
What technologies should we adopt?
When should we adopt them?
How should we adopt them?
I became fascinated by these questions about four years ago when I realized three things:
The lifelong stakes of our technology adoption decisions are huge
I was making the decision poorly
I could do way better with one mental model
#1: The lifelong stakes of our technology adoption decisions are huge
Over my life, I’ve faced many big tech adoption decisions:
Internet
Social media (as a consumer and creator)
Cryptocurrencies
Industry-specific tools (e.g., note-taking, writing, marketing)
AI
Etc.
As I’ve made these adoption decisions myself and seen my friends make them, I’ve seen the magnitude of the impact that this single decision can have on someone’s career.
For example, in a sinking field, there are fewer and fewer small opportunities at lower and lower pay. In a rising field that’s taking off like a rocket ship, there are more and more big opportunities at higher pay. For example, my friends who went into journalism generally ended up struggling while those who went into tech thrived financially.
Bottom line: As time goes by, tech becomes more important to all fields. As a result, the stakes of tech decisions are becoming faster and bigger.
#2: I was making the decisions poorly
As I saw different tech adoption choices play out, I saw two things:
My decision-making processes were falling into four buckets.
These four buckets led to vastly different results.
The quadrant below visually shows the decisions and their result:
My decisions were either unconscious:
Blind Early Adoption. I got wrapped up in hype cycles where I let my decisions be determined by clickbait headlines, slick demos, popular opinion, and my love of sci-fi tech rather than deep understanding. As a result of blind early adoption, I was years too far ahead of trends.
Blind Late Adoption. After wasting time on hype, I went to the other end of the spectrum. When new technologies came about, I instinctively flinched away and dismissed the technology out of hand without research or reflection. I just assumed that once the technology was good enough, others would tell me about it, and the downsides of this late adoption would be minimal.
Or the decisions were conscious:
Stubborn Resistance. Rather than flinching away, I would lightly keep on top of things, but I would wait for other people to figure out best practices, top tools, and trusted experts. Furthermore, I would wait for the price and quality to be good enough to deliver amazing results affordably. This approach was stubborn because I wasn’t considering the benefits of smart early adoption.
Smart Adoption. I now settle on the best path as being proactive and deliberate. More specifically, if you identify a technology that seems important and fundamental, it’s worth taking the time upfront to understand it deeply and then using that knowledge to make smart decisions based on reality rather than naivety or stubborness. Nearly anything is better than making major decisions haphazardly without deep understanding and deliberate reflection.
Bottom line: Through all of these experiences, I’ve realized that black-or-white thinking about tech adoption is a failing strategy. Rather, a better approach is dialectical thinking where I consider all of the pros/cons so I can get the benefits without most of the costs.
#3: I could do way better with one mental model
Over time, I realized that while the future is hard to predict, there is a lot we can do to understand how it might play out and to plan accordingly now.
More specifically, I learned that there was a simple and proven decision-making algorithm that almost all top innovators and futurists (Steve Jobs, Jeff Bezos, Elon Musk, Ray Kurzweil, Kevin Kelly) use:
Identify and understand important long-run exponential trends
Extrapolate those trends into the future
Think through the implications
Bottom line: Even if the exact future we predict doesn’t happen, it still helps us feel better and have better situational awareness and adaptability. Furthermore, the more we know, the more we can educate others in our niche about as an AI thought leader. Overall, the most practical thing you can first do with a huge technology adoption decision is to educate yourself.
Summary
Now you have a deeper understanding of the three big tech adoption insights I had:
The lifelong stakes of our technology adoption decisions are huge
I was making the decision poorly
I could do way better with one mental model
To help these lessons sink in, let me share a personal story that illustrates how I came to them…
My Personal Turning Point With AI Adoption
In July 2020, one of my closest and smartest friends visited my house. OpenAI had just released GPT-3 the month prior and throughout the few days he was with me, he kept on talking about:
How big of a release GPT-3 was
How it was going to change everything in a few years as it evolved
How important AI safety would be as a result
Even though I saw demos of the GPT-3, I discounted everything on a few premises:
There was no guarantee that it would become more useful in the near future. What if it hit a plateau I wondered.
Focusing on AI safety so early sounded like sci-fi and wasn’t practical.
Even though GPT-3 could do amazing things, it still made many silly errors.
On our final night together, I remember sitting around a campfire and encouraging him NOT to go all in on AI because it was too early and uncertain.
I didn’t think about AI again until November 2022, the month OpenaAI released ChatGPT, which anyone in the world could use for free.
Amazingly, just changing the interface with the same exact reasoning abilities from 2020 caused a huge wave of excitement, and over 100M+ signed up to pay for it within months, making it the fastest-growing product in human history.
On the one hand, it seems to have come out of nowhere. On the other hand, information about AI’s potential has already existed for two years. I could’ve gotten access to GPT-3 and played around with it. Also, the seminal academic paper on LLM Scaling Laws was published in January 2020. If I had read this paper, I would have had more confidence that the capabilities of AI would continue to improve.
In retrospect…
My #1 Lesson Learned In Retrospect
I shouldn’t have blindly rejected AI based on an uninformed first opinion.
What I know is that exponential trends go through two phases:
Deceptive
Disruptive
During the deceptive phase, the trend is growing exponentially, but it’s growing off of such a small base that it’s hard to feel its speed. Furthermore, the trend hasn’t gotten to a point where it’s actually useful. Therefore, it’s easy to overlook.
The deceptive phase is the optimal time to research the trend for a few reasons:
The are enough signals that demonstrate that the trend will continue.
There is enough time to prepare for the trend.
If there is a big opportunity, you can capitalize on it early.
If I had put in a relatively small amount of time, I would’ve more deeply understood GPT-3 capabilities/timelines/implications and been able to make an informed decision about what to do about AI.
This experience and several others like it have convinced me of the power of being proactive and deliberate about major technology decisions and then making smart decisions from there.
Now that you understand the importance of AI mental modeling along with proactive and deliberate decision-making on AI, let’s go another level deeper on understanding the AI Scaling Law, as it is arguably the most important model to learn first…
AI Scaling Law Breakdown
In AI Scaling Law Part 2, I break down the AI Scaling Law.
Below is the key chart from the key academic paper that introduced the scaling law.
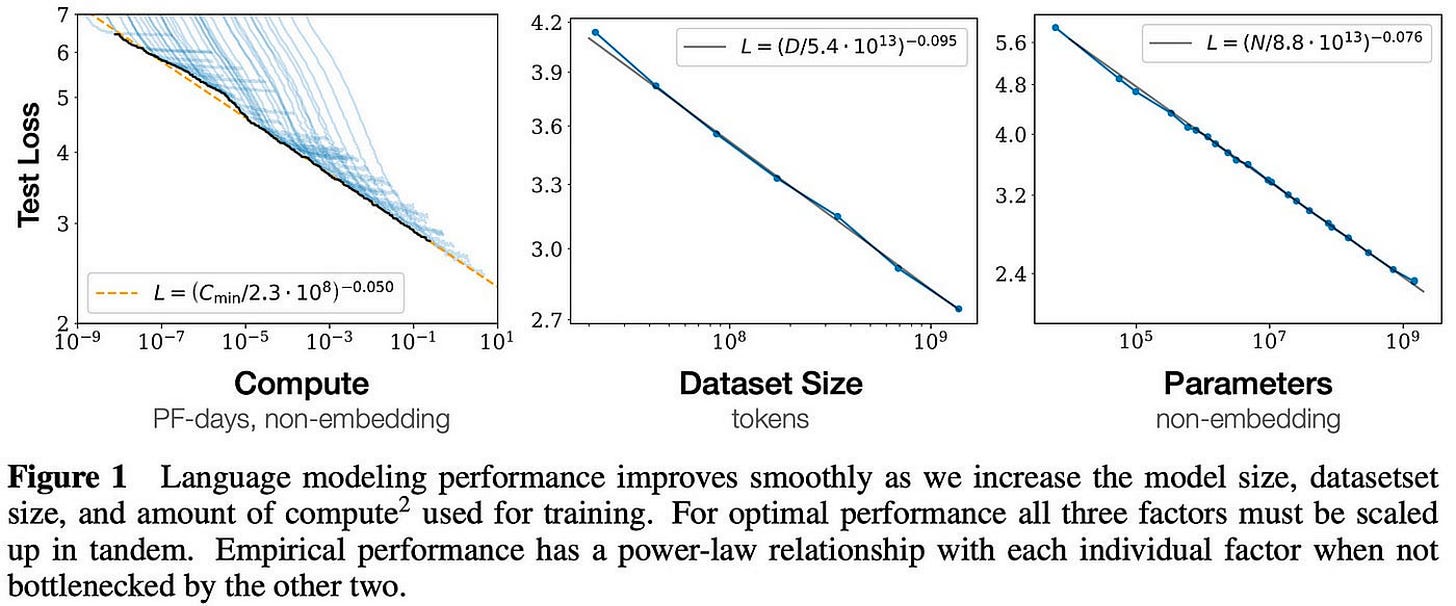
In short, the AI Scaling Law for LLMs (Large Language Models) is that performance very reliably increases as a function of three variables:
Compute
Data
Parameters
We can see this, for example, with the evolution of OpenAI’s GPT:
The AI Scaling Laws are important on two levels:
They increase our confidence that we can keep improving AI’s capabilities just by doing what we’re already doing, but more of it. This motivates companies to invest the necessary resources to increase AI’s capabilities.
The AI Scaling Laws help us predict the exact amount and proportion of data, compute, and parameters that will be needed in order to keep scaling AI’s capabilities…
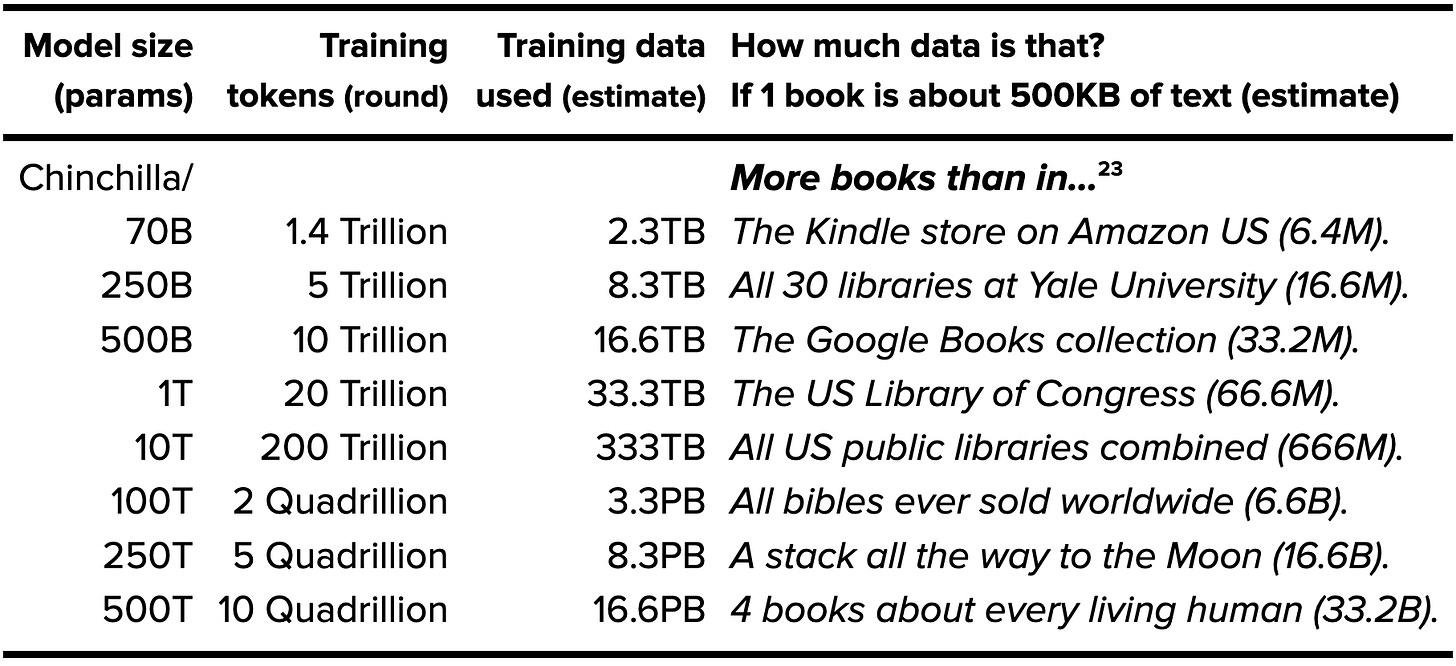
Another way to think of The AI Scaling Law is that it’s the modern version of Moore’s Law, except it’s much steeper.
In this post, I go down a level deeper than compute, data, and parameters, and I explore their parts. In other words, I make the model more granular.
It’s important to understand these parts because improving AI scaling doesn’t happen based on the rate of just one part. Each part builds on the others, and the system is only as strong as its weakest link.
Said differently…
These exponential trends stack on top of each other in terms of cost, money spent on compute, cost efficiency of compute with better computer chips, and improved algorithms, which means that the effective inputs into developing these systems are growing very rapidly.
—Tom Davidson, Researcher, Open Philanthropy
The Components Of AI Scaling
Software/Hardware Architecture
AI Workforce
Financial Investment
Raw Materials
Energy
Compute
Data
Algorithms
Prompting Techniques
Tool Use
#1. Software/Hardware Architecture
Neural nets
Deep learning
Large Language Models (LLMs) with unsupervised learning
Reinforcement learning from human feedback (RLHF)
GPU Chips (1999)
Transformers (2017)
Because the current architecture works so well, almost all of the money in the industry is focused on scaling it. At the same time, there are significant resources focused on new architectures or big innovations in the existing architecture (Mamba, Jepa, LPUs, Open-Endedness, Q*, Quiet-Star, Active Inference + Collective Intelligence, Continuous Learning).
In the video below, one of the top AI experts in the world, Andrej Karpathy, explains the pivotal impact that transformers had on the field:
Transformers: The best idea in AI | Andrej Karpathy and Lex Fridman
The Mamba architecture is interesting enough as a breakthrough technology that one of the AI explainers I respect the most did a whole emergency POD on the topic in early 2024:
#2. AI Workforce (talent, companies)
Quantity and quality of employees in the field
Entrepreneurs
AI Scientists
Prompt Engineers
AI Safety
Material Science Researchers
Quantity and quality of companies in the field
Foundational models
Toolmakers
#3. Financial Investment
The amount that's been spent in terms of dollars on the largest training runs has been increasing by about a factor of three over the last ten years, every single year.
—Tom Davidson
To put this in context, this would be a 59,049 increase over 10 years if it were to continue...
#4. Raw Materials
Computer chips are made up of various resources. I haven’t done much research yet on:
What those resources are
How plentiful they are
What promising alternatives are
#5. Energy (creation, distribution, storage, transformation)
Source: 2024 Bosch Connected World Conference
So over a year ago the shortage was chips. Then it was very easy to predict that the next shortage will be voltage step-down transformers. So because you've got to feed the power to these things. So if you've got 100 to 300 kilovolts coming out of your utility, and it's got to step all the way down to, you know, 0.6V, that's a lot of stepping down. Then the next shortage will be electricity. So I think next year you'll see the electricity that they just can't find enough electricity to run all the chips.
—Elon Musk
As de Vries explains in commentary published in Joule last year, Nvidia accounts for roughly 95 percent of sales in the AI market. The company also releases energy specs for its hardware and sales projections.
By combining this data, de Vries calculates that by 2027 the AI sector could consume between 85 to 134 terawatt hours each year. That’s about the same as the annual energy demand of de Vries’ home country, the Netherlands.
—The Verge
AI is the extractive industry of the 21st century… The amount of energy and water used for generative AI is somewhere between 1,000 to 5,000 times more than traditional AI.
—Kate Crawford, AI Scholar
At the World Economic Forum’s annual meeting in Davos, Switzerland, Altman warned that the next wave of generative AI systems will consume vastly more power than expected, and that energy systems will struggle to cope. “There’s no way to get there without a breakthrough,” he said.
—Kate Crawford, AI Scholar
#6. Data (human, synthetic)
Reinforcement learning from human feedback is a mechanism for using data from humans to kind of tweak the performance of a model like GPT-4 after it's already been trained on a huge amount of internet text.
There's a technique called constitutional AI that was developed by Anthropic, where AI models review their own outputs, score themselves along various criteria, and that, you know, is then used as data to improve that AI model.
—Tom Davidson, Researcher, Open Philanthropy
And there was recently a very large improvement in mathematical abilities of language models with a paper called Minerva, where the main thing they did is they just took a lot of math and science papers and they just cleaned up the data for those science papers so that previously certain mathematical symbols had not been correctly represented in the data. And so, you know, the data hadn't really shown language models how to do maths properly. They clean that data so that now all the symbols were represented correctly. And just from that data improvement, mathematics performance improved very dramatically.
—Tom Davidson, Researcher, Open Philanthropy
So you can imagine I is paraphrasing existing internet documents so that they're not exact repeats but maintain the meaning and then training on those already. There are papers where um, AI generates attempted solutions, for example, to a coding problem, and then those are checked kind of automatically. And then only the good solutions are then fed back into the training data that they will probably be lots of creative ways in which AI companies are trying to produce more high quality data. And increasingly, they'll be able to leverage, um, kind of capable AI systems to produce that. While AI systems are less capable than humans, there's going to be a limit there, because ultimately the data from the internet is coming from from humans. And so the data the AI is producing might be less lower quality. And there are also problems you get at the moment where if you continually train on data that you're producing, then progress does tend to stall, as I understand it from the papers I've read. But I think they'll be they'll be pushing on improving those techniques.
—Tom Davidson, Researcher, Open Philanthropy
#7. Algorithm (parameters, quality, efficiency)
The quality of algorithms and their efficiency have been again doubling every year.
—Tom Davidson, Researcher, Open Philanthropy
Shortly after GPT 3 was released, there was a turbo chat, GPT 3.5 that was released that was much faster and much more efficient in terms of the amount of compute that was used by OpenAI servers. And there are various techniques like quantization and flash attention, that just allow you to run a model with a very similar performance to your original model, but use less compute to do so, and so that that's again, you don't need additional data chips to benefit.
—Tom Davidson, Researcher, Open Philanthropy
#8. Compute (designers, manufacturers, suppliers)
The quality of the kind of cost efficiency of AI chips has been doubling every two years or so.
—Tom Davidson, Researcher, Open Philanthropy
The artificial intelligence compute coming online appears to be increasing by a factor of ten every six months. Like now, obviously that cannot continue at such a high rate forever, or it will exceed the mass of the universe. But, I've never seen anything like it. And this is why you see Nvidia's market cap being so gigantic. Because they currently have the best neural net chips. I mean, I think Nvidia's market cap exceeded the GDP of Canada or something recently. It was quite high. The chip rush is bigger than any gold rush that has ever existed.
—Elon Musk
Source: Cathie Wood at TEDAI
#9. Prompting Techniques (Chain of thought, few shot)
People may have heard of the prompt think step by step or chain of thought prompting where you just simply encourage a model and you give it a question like, you know, what's 32 times 43? And instead of outputting an answer straight away, you encourage it to think through step by step. So it does some intermediate calculations, um, and that can improve performance significantly on certain tasks, especially tasks like maths and logic that require or benefit from intermediate reasoning. There's other prompting techniques as well, like few shot prompting where you give the AI few examples of what you want to see that can significantly improve performance.
—Tom Davidson, Researcher, Open Philanthropy
#10. Tool Use
There's also been improvements driven by better tool use. There's a paper called Tool Former where they train a language model that was initially just trained on text. They train it to use a calculator and a calendar tool and an information database. And then it's able to learn to to use those tools. And actually ultimately, it kind of plays a role in generating its own data for using those tools. Then its performance again, as you might expect, improves on downstream tasks. GPT four if you if you pay for the more expensive version, you can you can enable plugins which allow, um, GPT four to use various tools like web browsing and music code interpreter to kind of run, um, code experiments.
—Tom Davidson, Researcher, Open Philanthropy
There’s a kind of class of techniques. I'm referring to scaffolding, where the AI model is kind of prompted to do things like check its own answer and find improvements, and then kind of have another go at its answer where it's prompted to kind of assign break the task down into subtasks, and then kind of assign it to those subtasks to another copy of itself, where it's prompted to kind of reconsider its high-level goal and and how its actions are currently kind of helping or not helping achieve that goal. That kind of scaffolding underlies, Auto GPT, which which people may have, may have heard of a kind of agent AI that is powered by GPT-4 and this scaffolding that kind of structures the GPT for thinking.
—Tom Davidson, Researcher, Open Philanthropy
Now that we understand many of the factors that affect AI's performance and scalability, we can begin to understand Musk’s comment about a fast timeline…
Four Ways Artificial Superintelligence Could Happen Much Faster Than We Predict
There are four fundamental reasons why AI could go faster than we think:
AI improves AI 100x faster
There’s a surprising breakthrough
Emergent and powerful capabilities emerge as model scales
AGI is attracting more and more attention
#1. AI improves AI 1,000x faster
Source: Tom Davidson, Senior Researcher at Open Philanthropy, on the Future Of Life Podcast
As these models' reasoning ability improves (e.g., GPT-4 → GPT-5), that reasoning ability can then be used to speed up the rate at which AI develops at every single one of the parts above (chip design, chip manufacturing, prompting, programming, energy, cleaning data, creating synthetic data, etc.). In other words, imagine hundreds of thousands of digital AI scientists who are just as smart as the smartest AI researcher but have a few extra superpowers:
They work 4x more. They can work every second of every day without distraction on AI research.
They have 1,000,000x more knowledge. They can get educated and remember all of humanity’s knowledge.
They work 1,000x faster. They are only limited by the speed of computers.
They’re much more scalable. As more compute comes online, each digital AI scientist could duplicate itself perfectly for a tiny fraction of the cost of scaling a human workforce.
They’re constantly getting faster, more knowledgeable, and smarter. At the point that they equal human intelligence, they will have more ability than ever to improve themselves.
#2. There’s a surprising breakthrough
We know that the current architecture is incredibly inefficient compared to what it could be. We know this because the efficiency of the human brain is proof that better is possible. Human brains can learn complex behaviors with just a few examples. Furthermore, they can do so with incredibly little energy.
Therefore, there could be new breakthroughs that vastly speed up progress that aren’t currently factored in. For example, the invention of transformers at Google in 2017 is a key breakthrough that has made the progress since then possible. Some are arguing that Mamba could be the next transformer.
While it is hard to predict when a new breakthrough will occur, it’s safe to assume that we increase the odds of this as a function of how much cumulative effort humanity puts into it. Given the stakes of AI, more and more resources are flowing into the next breakthrough.
#3. Emergent and powerful capabilities emerge as model scales
As new models are created, they develop new abilities. Therefore, a new model might develop a new ability that makes it vastly more useful and groundbreaking in ways we can’t predict in advance.
#4. AGI attracts more and more attention and resources
AGI is the biggest opportunity and threat in the history of business. The prize for being first is tremendous while the cost of being flatfooted is equally high.
Threefore, as AI becomes more useful and ubiquitous, companies invest more resources into it. As we start to see the light at the end of the tunnel where AI becomes AGI, there could be a huge increase in resources spent. For more on this phenomenon, read Meditations On Moloch. For a simpler introduction, I recommend this 12-minute video:
Conclusion: How To Think About Musk’s Prediction
Very few people are predicting that there will be an intelligence explosion to AGI in 2025 like Elon Musk.
At the same time, many top AI researchers do recognize that there is a possibility that it could happen.
This possibility should increase the urgency for all of us to start preparing now. Because even if there is a small chance that AGI could happen next year, the resulting impact would be so huge that it would be worth prepping for.
More broadly, by understanding the components that determine AI timelines, we can begin to understand factors that could cause the LLM Scaling Laws to continue, accelerate, or decelerate. I will explore these topics further in a soon-to-be-published post.
Appendix: Top Resources On Takeoff Speeds (Paid Subscribers)
Want to go deeper on the topic of AI timelines? After spending 100+ hours combing through the noise of the internet, I collected my 6 favorite resources on AI timelines for you…